What's the new dbt Cloud™ price increase about? - Part 2
In this article, we’ll walk you through everything you need to know about the new dbt™ pricing plan and what are your options.
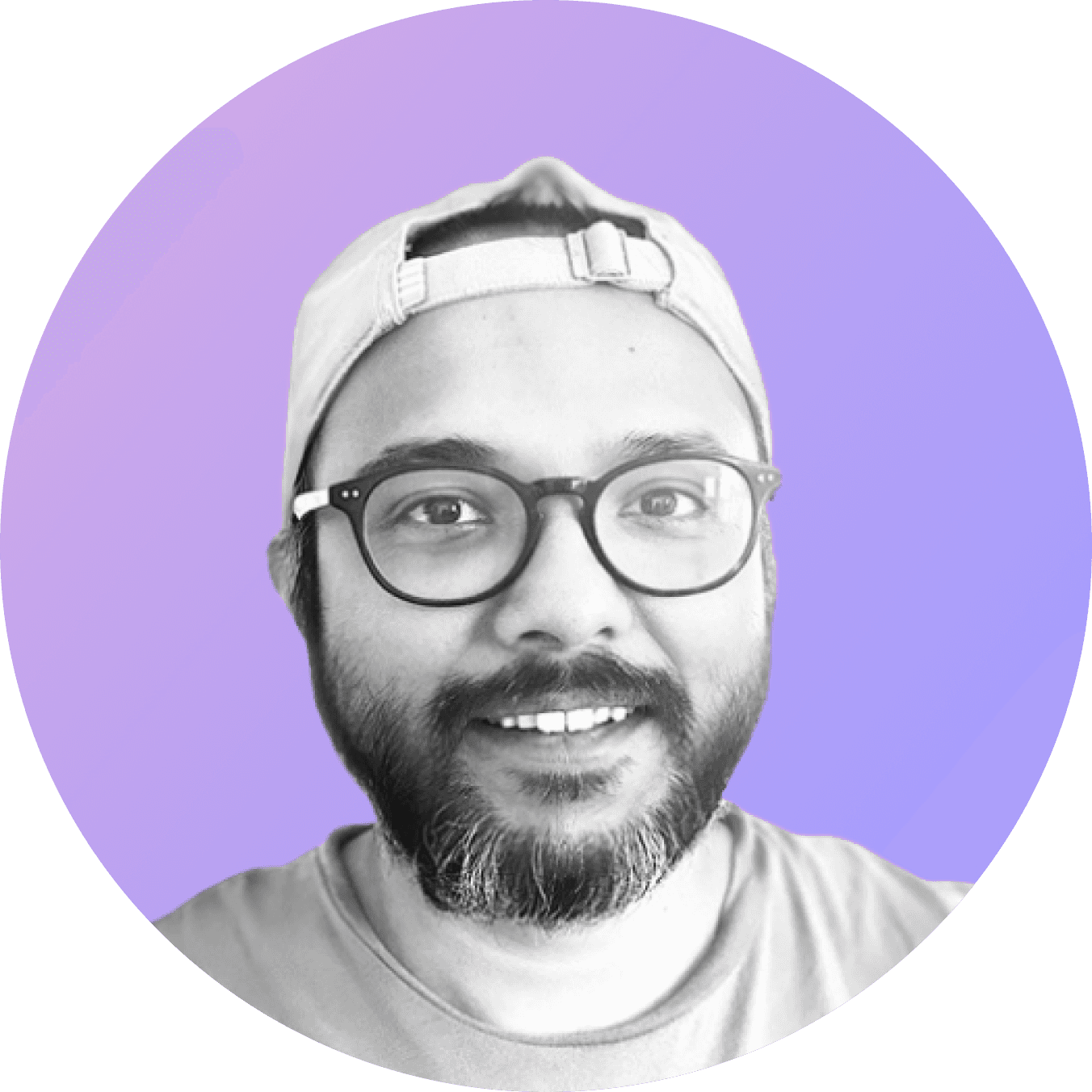
Kaustav Mitra
Jun 13, 2024
·
5
min read
Over the last 6 months, dbt Cloud™ pricing has changed drastically. On top of the 100–700% increase from Dec-2022, we are now looking at Shiny New Pricing that’s going to cut even deeper holes into already stretched analytics budgets.
In this article, we’ll walk you through everything you need to know about the new dbt™ pricing plan. We’ll help you answer questions like:
How does dbt™’s old price plan compare to the new one?
dbt™ is now charging per “successful model build”. What does that mean?
How will model-based pricing affect me?
What are the options on the table?
Why should you act now?
Let’s jump right into it by answering the first question:
1. How does dbt™’s old price plan compare to the new one?
Here’s a simple illustration of the old price plan vs the new one. Don’t worry, we’ll summarize it for you below.
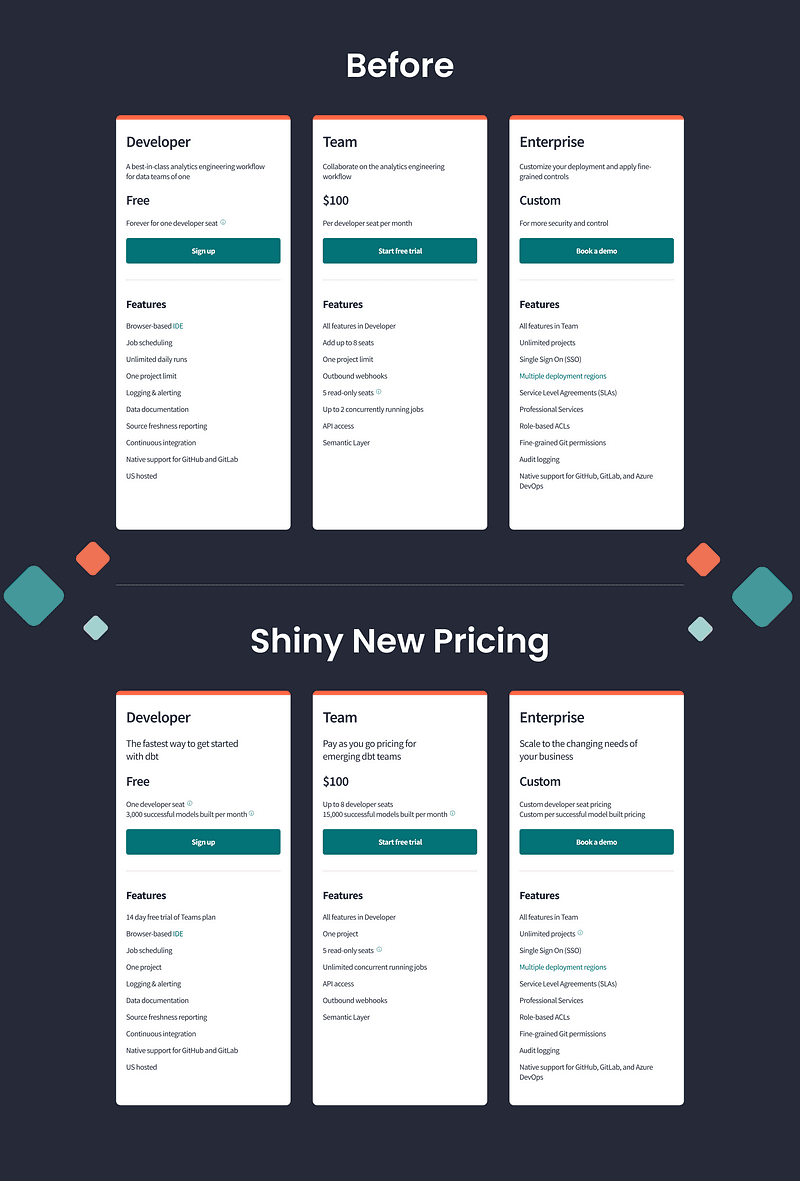
Developer Tier:
Before: Free for one seat. You could build as many models as you’d like at no extra charge
After: “Free” (emphasize the quotations 😂) for one seat. You will be able to run up to 3,000 model builds per month. More than that, you will need to pay $0.01 per successful model build. If you don’t pay, your models won’t run. 🚨If you have production jobs, they will stop running unless you pay.
Team Tier:
Before: $100 per seat. You could build as many models as you’d like at no extra charge
After: dbt™’s billing formula explains this new pricing clearly:
($100 x number of developer seats) + ((models built — 15,000) x $0.01)
Let’s say your organization has 12 seats and you run 95k models per month, how much will you be paying each month?
Before: ($100 x 12) = $1,200/month
After: ($100 x 12) + ((95,000–15,000) x $0.01) = $2,000/month.
Enterprise Tier:
Before: Prices vary (roughly $400/developer/month)
After: Whatever you were paying before the price increase + you will now pay additionally for model builds.
2. dbt™ is now charging per ‘successful model build’. What does that mean?
Let’s read dbt™’s definition and then unpack it.
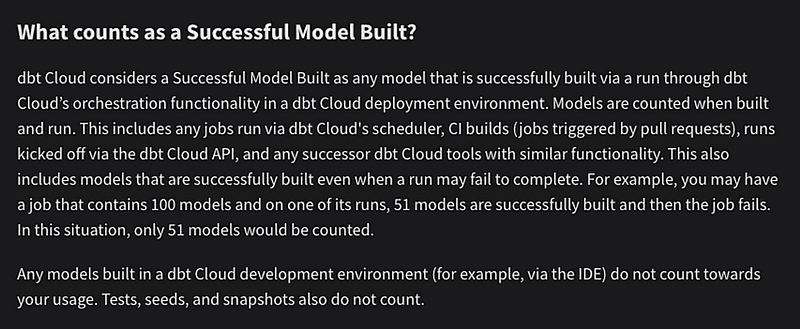
So, anytime you make a dbt™ run outside the dbt Cloud™ IDE environment (ex. a table and/or view is updated in your data warehouse), your dbt Cloud™ bill goes up by $.010 per successful model built. This price hike occurs whenever you execute any of the following:
Ad-hoc runs using the dbt Cloud™ scheduler
Automated dbt™ runs via your dbt™ Cloud scheduler
CI/CD builds in dbt Cloud™
Runs kicked off by through dbt Cloud™’s API
It does not matter if the whole run fails; all successfully built models during even a failed run are charged.
All of these dbt™ runs were free before the price hike.
3. How will model-based pricing affect me?
Let’s look at the impact of model build-based pricing. Financially, it comes down to how many models do you have and how many times do you run them during the day. But there is a deeper operational impact as well to consider.
A. Financial impact
To understand, how problematic this is, let’s say you have 500 models in the dbt™ repo.
If we assume:
a team of 8 developers
each developer makes ~4 pushes per day, so about 30 pushes overall
each push / CI-job runs 20% of models
there are 7 schedules / day that build 500 models each
all runs are successful (for simplicity)
These are pretty reasonable assumptions. We have users, who are running 100+ schedules.
With the above assumptions, we have:
from CI-jobs: 90,000 model builds/month [500 x 0.20 x 30 x 30]
from Production jobs: 105,000 model builds/month [7 x 500 x 30]
leading to a total of 195,000 model builds per month
In the team tier, with 8 seats, you would now be paying 225% more than before. Where your annual bill was $9.6k, it will now be around $31k!
B. Operational Impact
More than the financial impact, this situation presents a massive operational impact. I can immediately think of questions that would probably be top of mind for every data leader:
Will you be able to drive adoption of dbt™ across your organization?
Will you ask your team to build fewer models? That’s the equivalent of asking people to write fewer files of code, which is unheard of.
Will you stuff 600 lines of business logic into a single file to reduce model count? Aren’t we back in the pre-dbt™ days then?
Are you going to ask your analytics engineers to push less? They are having a hard time learning git already. Do you think this will improve their productivity?
Will you run production pipelines less? So stale data for everyone in the business.
Will you be constantly refactoring models to stay within budget? Will you keep breaking your dashboards and reports. Will that be good for your team morale or for your stakeholder relationships?
Will you go to finance every 2 months asking for a new budget? Aren’t they already asking you to reduce your warehouse spend?
These are real operational questions that data leaders need to ask. And whether the operational overhead for all of this is really worth it.
Depending on your team size, repo size, and scheduling frequency, you could be looking at your cost to increase between 160% and 1700%+.
From our estimates, if you have 200 models, then you can run ONLY 1 schedule and 1 CI job per day to keep your budget unaffected. That for all practical purposes is impossible to achieve.
4. What are the options on the table?
At the beginning of this year, Christof Blefari wrote a pretty insightful post on how to manage and schedule dbt™. At the end of the post he had a comparison chart, which I have re-produced below on all the various options available.
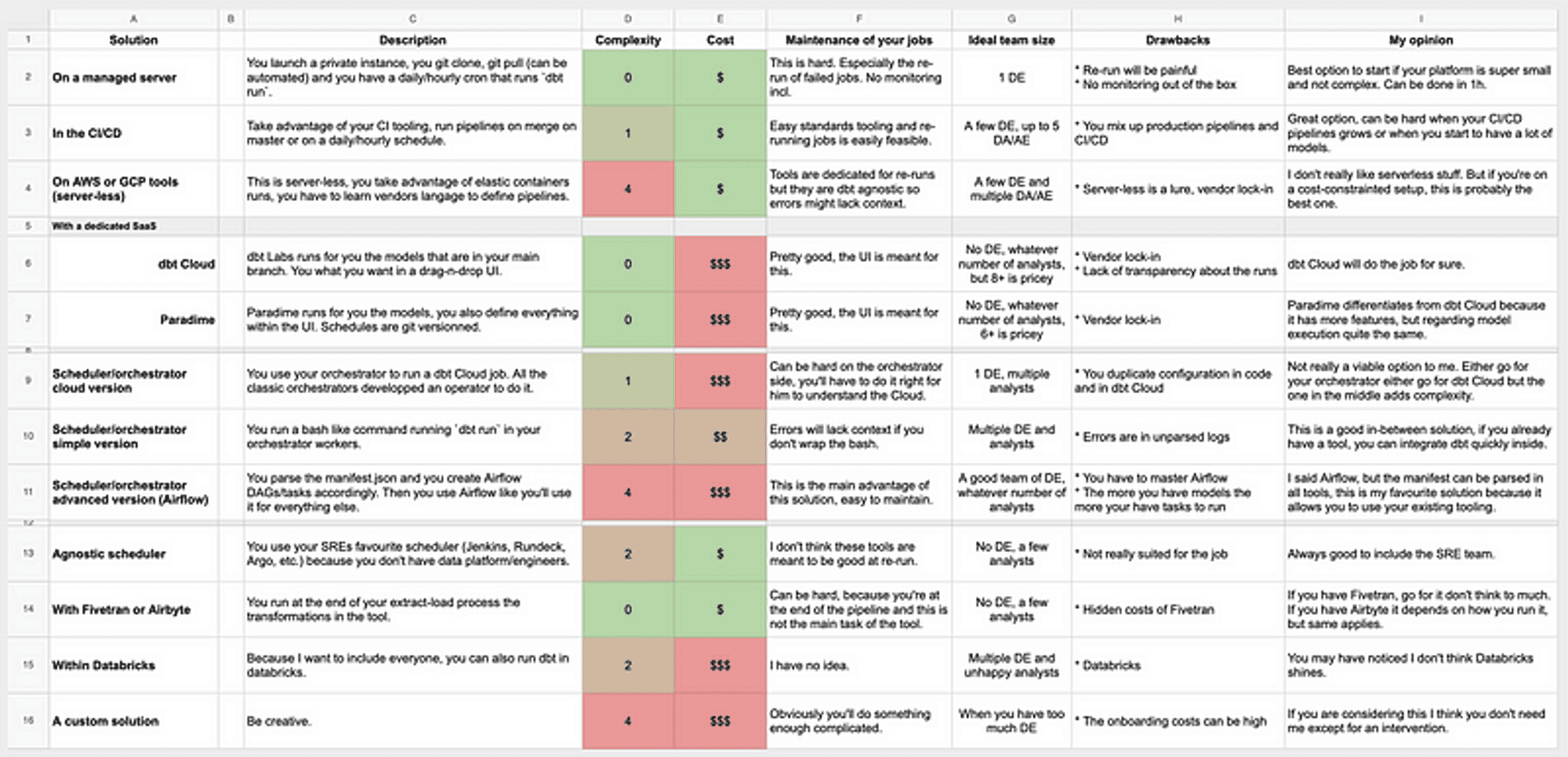
All the non-SaaS options, require both data engineering time and data engineering teams of varying sizes. Now, data engineering time is precious and it’s expensive. I would argue, its best spent solving hard data engineering problems than maintaining analytics platforms.
But in a non-SaaS world, you don’t have that option. So we created a little comparison to show what’s the actual cost of various options outside dbt Cloud™.
We assumed:
a team of 8 developers
each developer makes ~4 pushes per day, so about 30 pushes overall
each push / CI-job runs 20% of models
there are 7 schedules / day that build 500 models each
all runs are successful (for simplicity)
Based on the above, there would be 195,000 successful model-builds per month, and the alternatives and their associated costs would be:
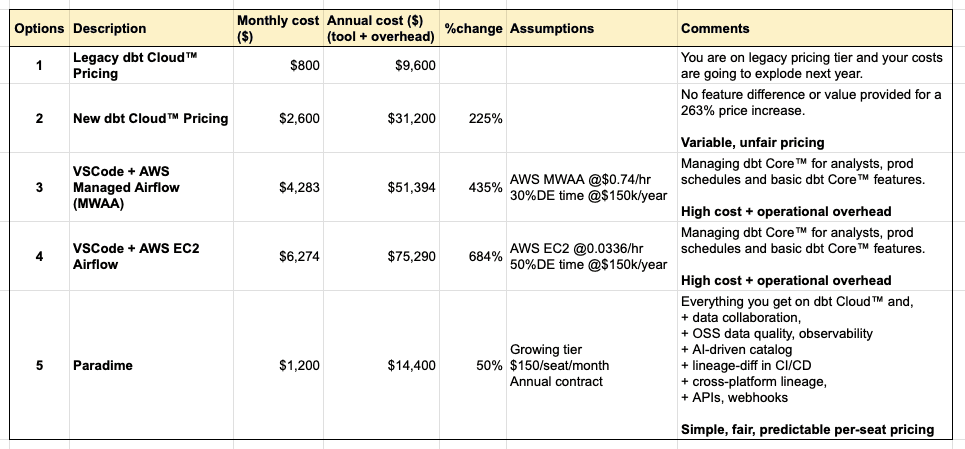
We can see that:
Sticking to the status quo means risking significant budget overruns, like paying 225% more in the above example.
Moving to a highly operationally intensive VSCode + dbt Core™ + Airflow solution, will be 4–6 times more expensive — considering both tool and personnel costs.
And, lastly, moving to Paradime, would get you everything you already need and additional features at a reasonable, fair, and predictable per-seat pricing.
5. Why should you act now?
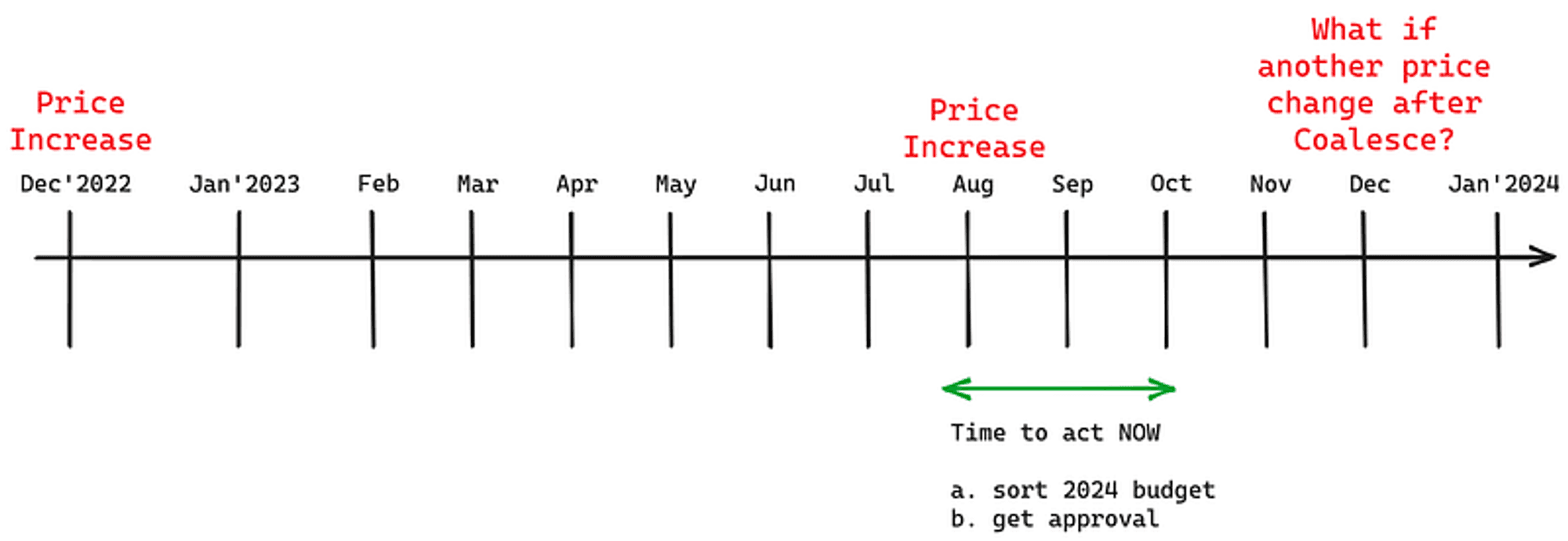
If we plot the timeline between Dec-2022 and Dec-2023, for a lot of teams, Jan-2024 will be the start of the new financial year. The time to get those budget approvals is between now and October. Otherwise, you run the risk of scrambling for a budget once again, just like what happened last year.
Making sure data budgets are flat from last year is top of mind for every CFO or VP Finance I have spoken to. It’s important that they are onboard with your plans.
As a thought exercise, how are you thinking of protecting / hedging yourself from another price increase in December? Paradime aside, I would love to know and help in any way I can.
I am also wondering, what if dbt™ Labs says, you will have to pay for model execution time as well after Coalesce?
Conclusion
In conclusion, if you are a data leader, and you are using dbt™ or considering dbt™ and reading this, you have three choices:
Use dbt Cloud™, which’s expensive, lacks features, and comes with variable and unpredictable pricing.
Opt for open-source with dbt Core™ with very-high personnel cost and operational overload attached.
Go for Paradime with a dbt™ native, easy-to-use platform for all your analytics work with smart, fair, and predictable pricing. Plus, if you are already using dbt™, migration takes minutes.
Sign up for a FREE 14-day trial or schedule some time with our team to learn more about Paradime 💪